Computational Imaging
To be updated!
Before 2017
- Direct Superresolution Technique for Realistic Image Reconstruction
- Computational Depth-Based Deconvolution Technique for Full-Focus
The goal of computational imaging is to extract additional information through advanced image processing algorithms. We have developed computational techniques to improve the image quality over a large depth of field and improve the image resolution over a large field of view. We have also working on superresolution techniques, including structured illumination microscopy and Fourier ptychography.
Direct Superresolution Technique for Realistic Image Reconstruction
Traditional superresolution (SR) techniques employ optimizers, priors, and regularizers to deliver stable, appealing restorations even though deviating from the real, ground-truth scene. A non-regularized direct SR technique, illustrated in Fig. 1, has been developed to uniquely solve a set of linear equations representing the multi-shift image reconstruction problem with sufficient measurements to deliver realistic reconstructions without any inherent bias imposed by improper assumptions in the inverse problem.
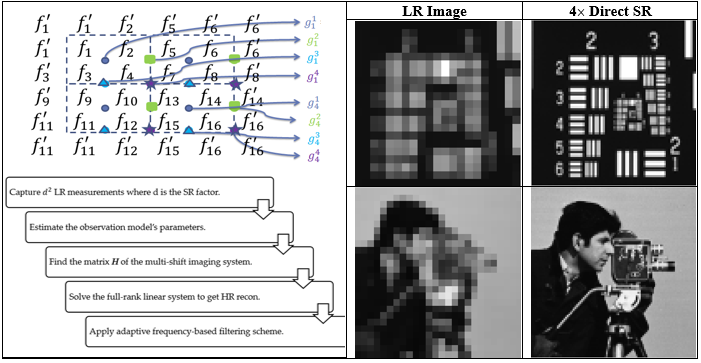
Fig. 1. The multi-shift LR measurements (top-left) and steps (bottom-left) summarizing the direct SR technique. A sample of low-resolution (LR) images and the direct SR’s reconstruction results at 4subsampling factor under ideal imaging conditions are shown for USAF target (top-right) and cameraman image (bottom-right).
An adaptive frequency-based filtering scheme (AFFS), shown in Fig. 2, is introduced to gain robustness against poor focus, misestimated shift, boundary variations, and noisy scenarios while maintaining the merit of direct SR to achieve optimal restorations with minimal artifacts.
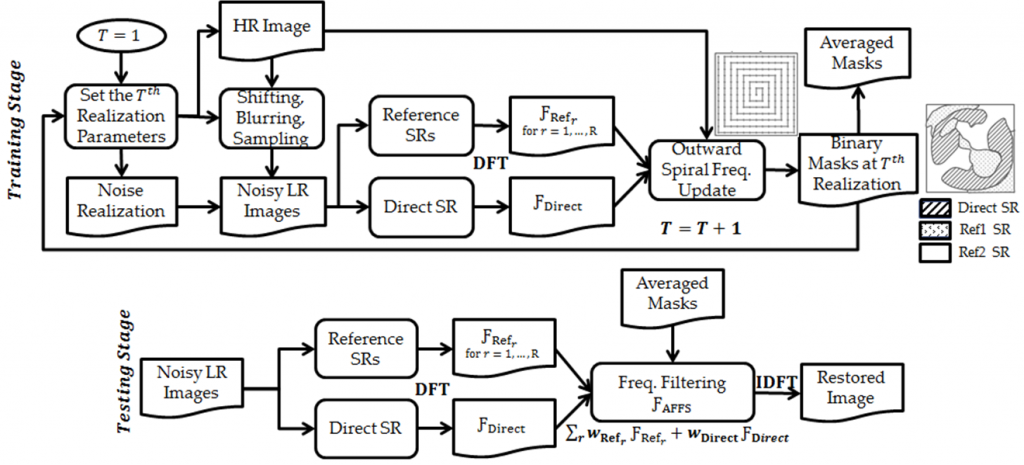
Fig. 2. Block diagram illustrating the adaptive frequency-based filtering scheme in the training stage (top) and the testing stage (bottom).
Simulation results, presented in Fig. 3, demonstrate that more fine features can be resolved with the developed technique as compared with other SR techniques such as bicubic SR and multi-channel blind deconvolution (MCBD) SR. Simulations also show that the retrieved high-resolution features are successfully transferred by the AFFS to produce an artifact-suppressed high-resolution image under realistic imaging conditions such as noisy environment, blurring capturing, boundary tolerance, and shift misestimate.
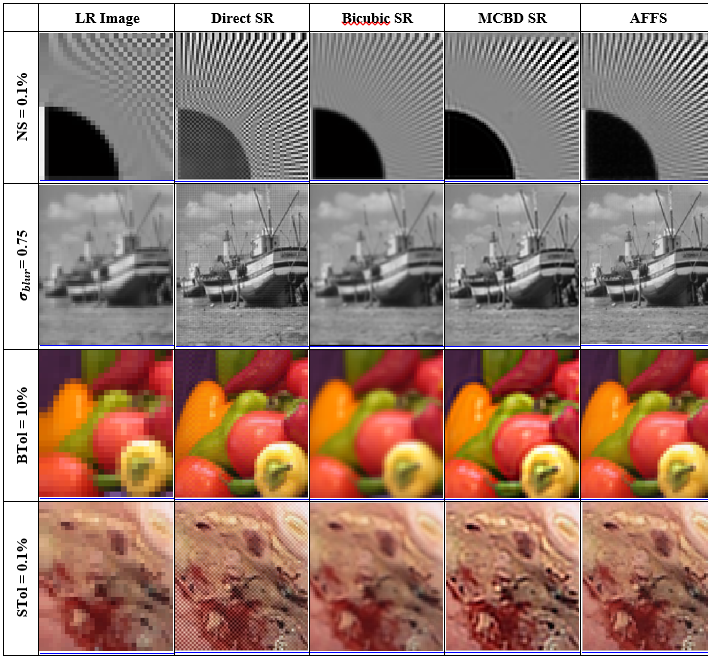
Fig. 3. Visual comparison of various SR techniques sorted row-wise at 2× subsampling factors under various realistic imaging conditions sorted column-wise using sensor shift model, noiseless environment (NS = 0 %), in-focus measurements (= 0.5 pixel), matched boundary conditions (BTol = 0%), and known non-integer-pixel shifts (STol = 0%) unless mentioned differently in each column. The associated numerical error RMSE% is shown at their left.
Publications
- B. Salahieh, J. J. Rodriguez, and R. Liang, “Direct superresolution for realistic image reconstruction,” Optics Express (OSA, 2015).
- B. Salahieh, J. J. Rodriguez, R. Liang, “Direct Superresolution Technique for Solving a Miniature Multi-Shift Imaging System,” in Imaging Systems and Applications, JW3A. 5 (OSA, 2015).
Computational Depth-Based Deconvolution Technique for Full-Focus
Ordinary fixed-focus cameras, such as low-end hand-held cameras, smartphone cameras, and surveillance cameras, have a depth-variant point spread function (PSF) that can be visualized as double cones when focusing at finite distances or as a single cone when focusing at infinity with apex at the on-axis in-focus point located at the hyperfocal distance. Objects become progressively more defocused as their distance to the lens gets smaller than half of the hyperfocal distance. The prior knowledge of the volumetric PSF can be utilized effectively to deblur the captured image at different depth planes.
A computational depth-based deconvolution technique, presented in Fig. 1, has been developed to equip ordinary cameras with an extended depth of field capability. This is achieved by deconvolving the captured scene with pre-calibrated depth-variant point spread function profiles to bring it into focus at different depth planes. Afterward, the focused features from the depth planes are stitched together to form a full-focus image.
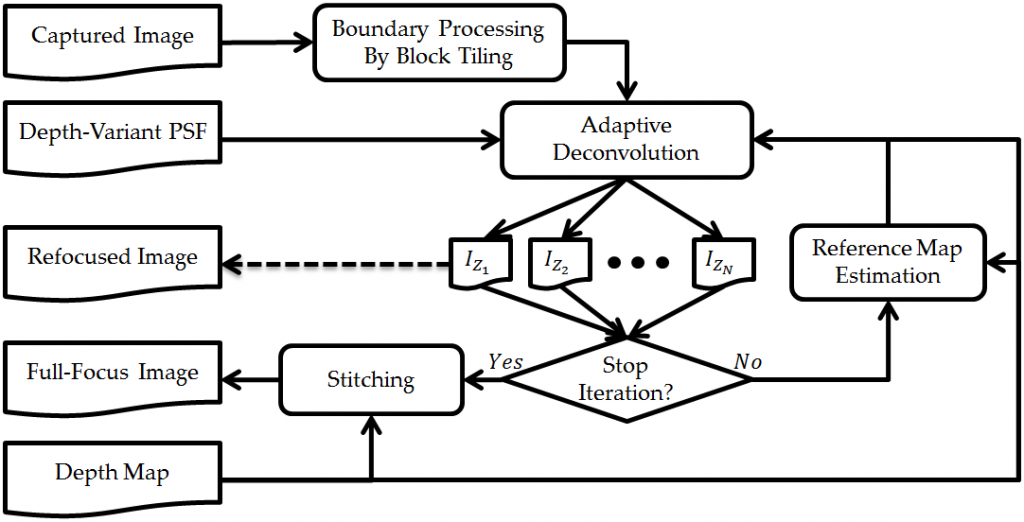
Fig. 1. Block diagram of the computational depth-based deconvolution technique
To imitate multi-depth (MD) objects, different regions of planar objects traced at various depth planes are stitched together according to a customized depth map, presented in Fig. 2.
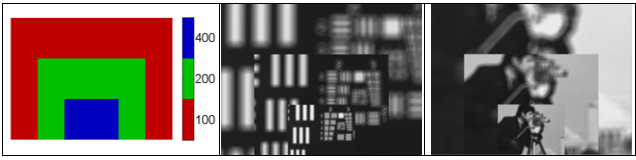
Fig. 2. Stitching planar regions according to a depth map (left) to form MD objects for USAF (center) and cameraman (right).
The ringing artifacts associated with the deconvolution process are addressed on three levels. First, the boundary artifacts are eliminated by adopting a block-tiling approach. Second, the sharp edges’ ringing artifacts are adaptively controlled by reference maps working as local regularizers through an iterative deconvolution process. Finally, artifacts initiated by different depth surfaces are suppressed by a block-wise deconvolution or depth-based masking approach. The developed algorithm is demonstrated for planar objects and multi-depth objects scenarios.
Note that the depth-transition artifacts can dominate the MD deconvolution results if no special processing is involved (see second column of Fig. 3). The depth-based masking approach can help a lot in reducing such artifacts (see last column in Fig. 3), although it is not fully restoring some of the image details (e.g., compare the vertical bars on the right of restored USAF images between the depth-based masking case and reference MD case).
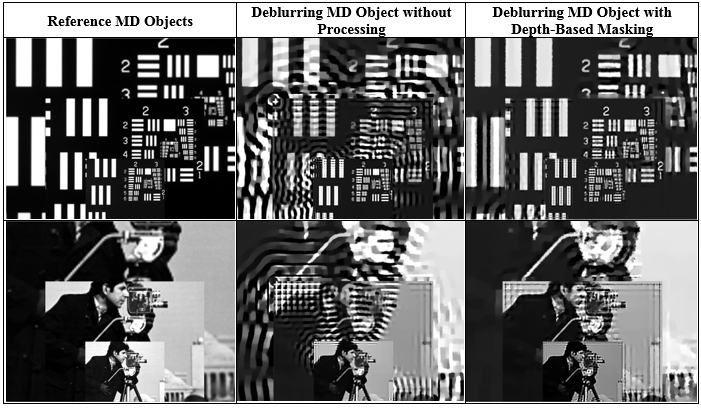
Fig. 3. Visual comparison of final full-focus deconvolution results after stitching based on a depth map for USAF and cameraman images showing reference MD objects (first column), deblurring results of MD object without any depth processing (middle column), and deblurring results of MD object with depth-based masking approach (last column).
Publications
- B. Salahieh, J. J. Rodriguez, S. Stetson, and R. Liang, “Single-image extended-focus technique using depth-based deconvolution”, submitted for publications (OSA, 2015).
- B. Salahieh, Jeffrey J. Rodriguez, and Rongguang Liang, “Computational Depth-Variant Deconvolution Technique for Full-Focus Imaging, ” Computational Optical Sensing and Imaging, (OSA, 2015).